The Importance of Objectivity in AI
Memory Matters #2
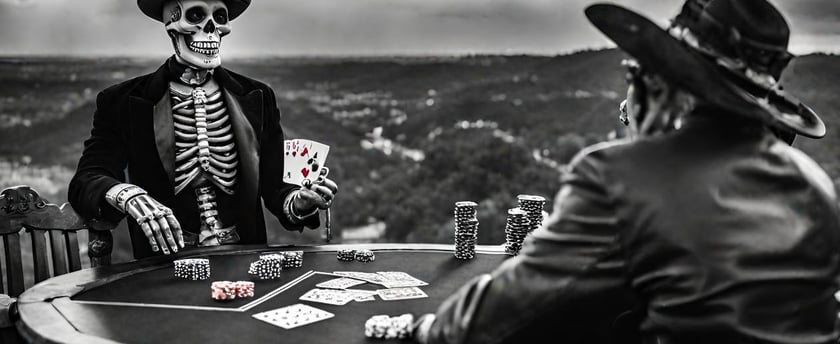
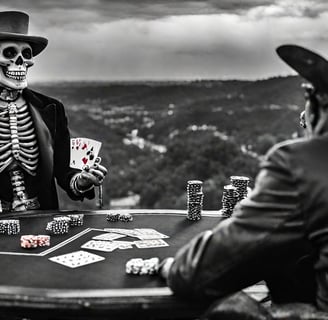
Objectivity in artificial intelligence (AI) pertains to the characteristic of being impartial, unbiased, and based on true information. This principle is crucial as it guarantees that AI systems carry out their functions relying on logical reasoning and empirical data rather than subjective judgments or preconceived notions. Objectivity is comprised of three essential elements: impartiality, fairness, and the absence of biases. Knowing that based on the algorithmic selection of use, biasing is common, these components are vital for the ethical and efficient use of AI technology.
Impartiality in AI development means ensuring that the algorithms and models do not exhibit any bias towards specific groups or outcomes. Algorithmically this is a difficult feat. Ensuring impartiality necessitates the implementation of thorough testing and validation procedures to identify and address any inadvertent biases that may emerge from the data or the algorithms' design. Fairness, which is closely associated with impartiality, involves ensuring that all users and stakeholders in AI systems receive equal and reasonable treatment. This idea necessitates that AI developers take into account the varied ramifications their technologies may have on different demographic groups and make efforts to mitigate inequalities.
Ensuring impartiality in AI is arguably the most difficult component of upholding objectivity. Biases can arise from multiple sources, such as the datasets utilized for training AI models, the assumptions made during the development phase, and the interpretation of the outcomes. For example, if an artificial intelligence system is educated with a dataset that accurately represents past preconceptions, it may continue to uphold similar biases while making decisions. Hence, it is crucial to employ varied and inclusive datasets and consistently assess the AI systems for any indications of bias.
The importance of objectivity in AI cannot be exaggerated. Objective AI systems have the ability to create decisions that are highly dependable, transparent, and trustworthy, which in turn enhances user confidence. Potentially could this be a post-processing type of task after the data is crunched? On the other hand, the absence of impartiality might result in adverse outcomes. For instance, AI systems with bias in recruiting procedures may unjustly put certain groups at a disadvantage, while predictive policing algorithms with bias could disproportionately focus on underprivileged communities. A human touch is always needed. These situations emphasize the crucial importance of prioritizing objectivity in AI to guarantee ethical and fair results.
Strategies for Achieving Objectivity in AI Models
Ensuring impartiality in AI models necessitates a multidimensional effort that demands a methodical and all-encompassing strategy. Using varied and inclusive datasets is one of the fundamental tactics is utilizing datasets that are varied and inclusive. To decrease the danger of biases arising from homogeneous data, AI developers can incorporate a diverse range of data sources that encompass distinct populations and scenarios. This method not only increases the model's fairness, but also promotes its ability to perform well and be reliable in many situations.
Incorporating rigorous testing and validation procedures is another essential approach to attaining objectivity. Thorough testing procedures, such as cross-validation and blind testing, aid in the early detection and correction of biases during the development process. These procedures guarantee that the AI model maintains consistent and fair performance across different subsets of data. Furthermore, it is crucial to consistently monitor and audit AI systems in order to identify and rectify any developing biases that may arise over time. Deploying the model ensures its objectivity.
Transparency and explainability are crucial to guaranteeing the impartiality of AI. Thorough documentation of the AI model's development, which includes information on the data sources, algorithmic decisions, and validation techniques, promotes transparency and responsibility. Transparent communication regarding the decision-making procedures of AI systems can facilitate stakeholders' comprehension and confidence in the results, hence diminishing the probability of biased interpretations. Transparency also enables external evaluations and audits, which are essential for identifying and reducing biases.
Objective AI models necessitate interdisciplinary collaboration, as well as ethical considerations. Collaborating with specialists from many disciplines, including sociology, psychology, and ethics can offer helpful perspectives on possible biases and how to address them. By adopting a collaborative approach, the AI development process takes into consideration a diverse range of opinions, thereby improving the model's objectivity. Moreover, by following ethical principles and frameworks, it guarantees that the AI systems are in line with societal values and norms, thereby fostering fairness and equity.
Linked to ObjectiveMind.ai for increased content distribution.